Oke OA, Cavus N. A systematic review on the impact of artificial intelligence on electrocardiograms in cardiology. International Journal of Medical Informatics. 2025 March 9:105753.
Electrocardiography (ECG) remains a cornerstone diagnostic tool in cardiology, and recent advancements in artificial intelligence (AI) have dramatically expanded its potential. This systematic review investigates how machine learning and deep learning models are being applied to analyze ECG signals, improving the accuracy, speed, and automation of cardiovascular disease diagnosis.
The study explores a wide range of supervised learning methods, from conventional algorithms such as k-nearest neighbors and support vector machines to more complex deep learning architectures like convolutional neural networks (CNNs), recurrent neural networks (RNNs), and long short-term memory (LSTM) models. Each technique’s strengths and limitations are evaluated in the context of arrhythmia detection, myocardial infarction classification, and heart failure risk prediction.
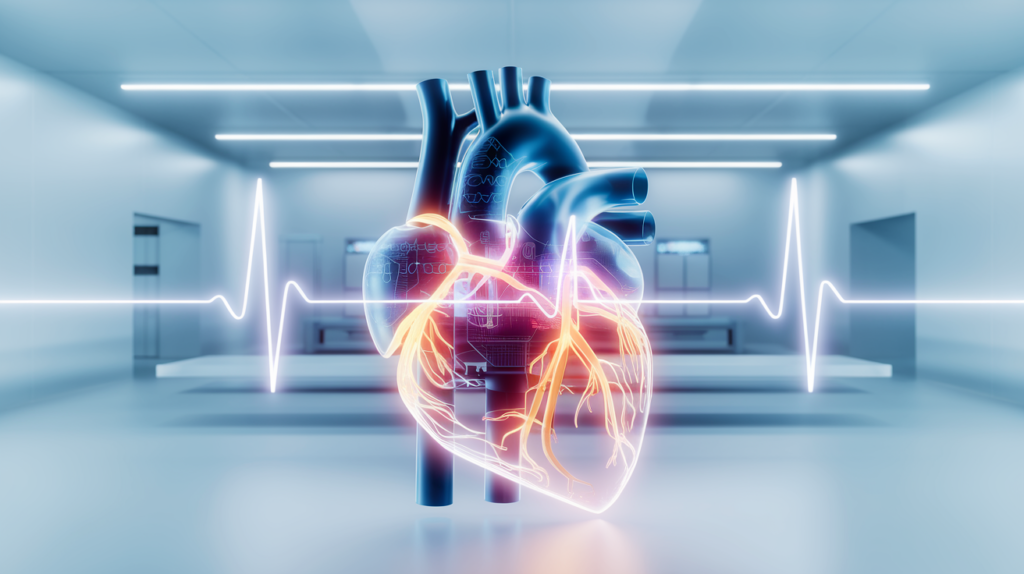
One of the key findings is the superior performance of deep learning approaches, particularly CNN-based models, in feature extraction and classification tasks without the need for extensive pre-processing. The review also highlights hybrid models and ensemble methods, which integrate multiple algorithms to improve generalizability and robustness across diverse datasets.
The study pays special attention to challenges such as noise in ECG signals, class imbalance in medical datasets, and the need for interpretability in clinical decision-making. By addressing these issues, the authors emphasize the importance of balancing model performance with practical implementation in healthcare environments.
This review provides a valuable roadmap for researchers and clinicians aiming to integrate AI-powered ECG analysis into routine practice. It also outlines future directions for improving model transparency, expanding clinical datasets, and enhancing cross-device compatibility in real-world applications.
About the researcher
Prof. Dr. Nadire Çavuş is a lecturer at the Faculty of Artificial Intelligence and Informatics at Near East University. She is also a researcher at the Computer Information Systems Research and Technology Center, where her work focuses on AI applications in health informatics, educational technologies, and intelligent systems. She welcomes collaborative research opportunities and can be reached at nadire.cavus@neu.edu.tr.
Abstract
Background: Artificial intelligence (AI) has revolutionized numerous industries, enhancing efficiency, scalability, and insight generation. In cardiology, particularly through electrocardiogram (ECG) analysis, AI has the potential to improve diagnostic accuracy and reduce the time needed for diagnosis. This systematic review explores the integration of AI, machine learning (ML), and deep learning (DL) in ECG analysis, focusing on their impact on predictive diagnostics and treatment support in cardiology. Methods: A systematic literature review was conducted following the PRISMA 2020 framework, using four high-impact databases to identify studies from 2014 to -2024. The inclusion criteria included English-language journal articles and research papers that focused on AI applications in cardiology, specifically ECG analysis. Records were screened, duplicates were removed, and final selections were made on the basis of their relevance to AI-ECG integration for cardiac health.
Results: The review included 46 studies that met the inclusion criteria, covering diverse AI models such as CNNs, RNNs, and hybrid models. These models were applied to ECG data to detect and predict heart conditions such as arrhythmia, myocardial infarction, and heart failure. These findings indicate that AI-driven ECG analysis improves diagnostic accuracy and provides significant support for early diagnosis and personalized treatment.
Conclusions: AI technologies, especially ML and DL, enhance ECG-based cardiology diagnostics by increasing accuracy, reducing diagnosis time, and supporting timely interventions and personalized care. Continued research in this area is essential to refine algorithms and integrate AI tools into clinical practice for improved patient outcomes in cardiology.
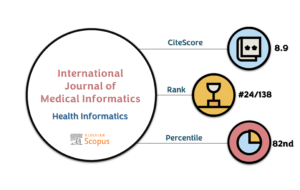