Kibcak E, Buhara O, Temelci A, Akkaya N, Ünsal G, Minervini G. Deep Learning-Driven Segmentation of Dental Implants and Peri-Implantitis Detection in Orthopantomographs: A Novel Diagnostic Tool. Journal of Evidence-Based Dental Practice. 2025 Mar 1;25(1):102058.
This study presents a deep learning-based approach designed to segment dental implants and detect peri-implantitis in orthopantomographs (OPGs). Peri-implantitis, characterized by inflammation and bone loss around dental implants, is a major cause of implant failure, making early detection crucial. Traditional diagnostic methods can be subjective and time-consuming; this research addresses these limitations with an AI-powered system offering enhanced consistency and efficiency.
The study utilized a dataset of 7,696 OPG images obtained from a university clinic and a private practice. Following exclusion criteria, manual segmentation of implants was performed by specialists using annotation tools, and a U-Net architecture was employed to segment implant regions. For classification, the AlexNet model was trained to distinguish between healthy and diseased implants based on image features.
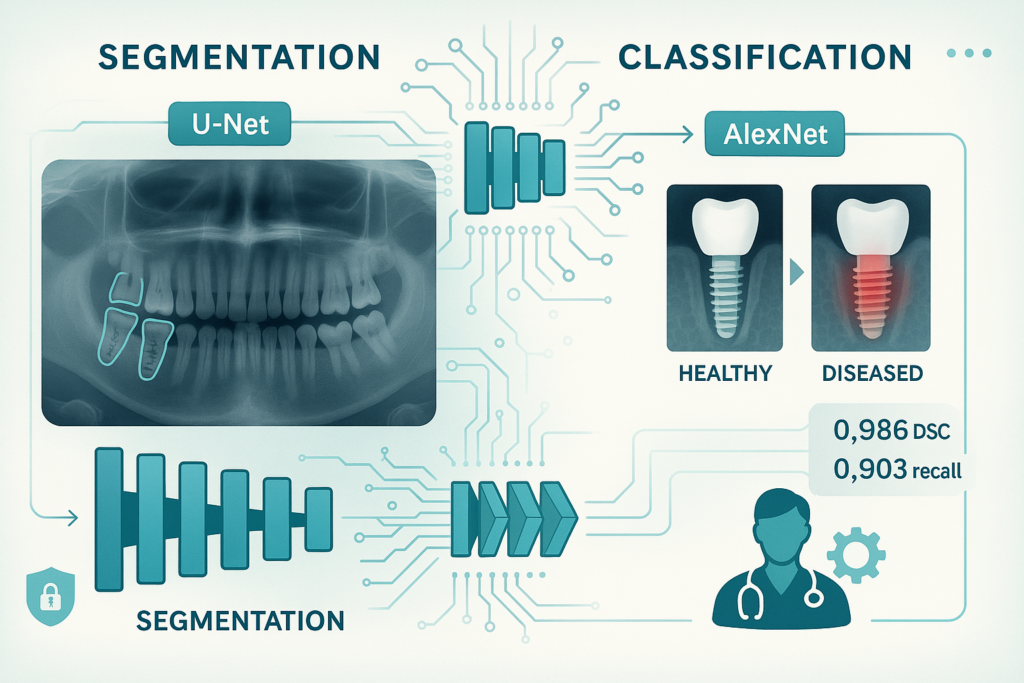
The segmentation model achieved a test accuracy of 0.999 and a Dice Similarity Coefficient (DSC) of 0.986. In classification, the model demonstrated a recall of 0.903 and an F1 score of 0.835, showing strong performance in detecting peri-implantitis. However, the study also acknowledges a relatively high number of false positives, highlighting the need for refinement before widespread clinical use.
The findings suggest that deep learning can assist clinicians in identifying early signs of peri-implant disease, potentially improving treatment planning. Still, ethical considerations such as data privacy and avoiding over-reliance on AI predictions are emphasized. Future work will focus on improving model precision and integrating the tool into real-world clinical workflows.
About the researchers
This collaborative study includes researchers from Near East University (Departments of Oral and Maxillofacial Surgery and Computer Engineering), along with international contributors from Western University (Canada), Saveetha University (India), and University of Campania (Italy). Assoc. Prof. Dr. Oğuz Buhara is a maxillofacial surgeon at the Near East University Faculty of Dentistry. He is open to academic collaborations in areas related to oral implantology and AI applications in dentistry, and oral surgery. He can be contacted via his institutional email address (oguz.buhara@neu.edu.tr).
Abstract
Introduction and Objective: Dental implants are well-established for restoring partial or complete tooth loss, with osseointegration being essential for their long-term success. Peri- implantitis, marked by inflammation and bone loss, compromises implant longevity. Current diagnostic methods for peri-implantitis face challenges such as subjective interpretation and time consumption. Our deep learning-based approach aims to address these limitations by providing a more accurate and efficient solution. This study aims to develop a deep learning-based approach for segmenting dental implants and detecting peri-implantitis in orthopantomo- graphs (OPGs), enhancing diagnostic accuracy and efficiency.
Materials and Methods: After applying exclusion criteria, 7696 OPGs were used in the study, which was ethically authorized by the Near East University Ethics Review Board. Using the Python-implemented U-Net architecture, the DICOM-formatted images were segmented and converted into PNG files. The classification model used a con- volutional neural network (CNN) for distinguishing between healthy implants and those affected by peri-implantitis, leveraging features extracted from the segmented regions to enhance diagnostic accuracy. The model was trained for 500 epochs using the Adam optimizer, with the dataset split into training (70%), validation (15%), and test (15%) sets. Dice similarity coefficient (DSC) and accu- racy were used to assess segmentation performance. Three medical profession- als used precision, recall, and F1-score to assess the classification model after segmentation, which determined whether implants were showing signs of peri- implantitis.
Results: The segmentation model achieved a test accuracy of 0.999, Dice Similarity Co- efficient (DSC) of 0.986, and Intersection over Union (IoU) of 0.974. For classification, out of 3693 implants, 638 were clinically identified as having peri-implantitis. The model correctly identified 576 of these, with 165 false positives. Performance metrics included a precision of 0.777, recall of 0.903, and F1-score of 0.835.
Conclusion: The deep learning-based approach for segmentation and classification of dental implants and peri-implantitis in OPGs is highly effective, providing reliable tools for enhancing clinical diagnosis and treatment planning.
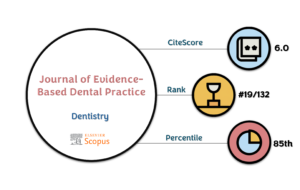