Usman AG, Aitani A, Usman J, Abba SI, Alhooshani K, Tanimu A. Optimization algorithms for modeling conversion and naphtha yield in the catalytic co-cracking of plastic in HVGO. Process Safety and Environmental Protection. 2025 April 26:106958.
This study focuses on modeling and optimizing the conversion efficiency and naphtha yield in the catalytic co-cracking of low-density polyethylene (LDPE) with heavy vacuum gas oil (HVGO)—a promising route toward reducing plastic waste and enhancing refinery efficiency. The research was conducted using a fixed-bed microactivity test (MAT) unit and explored various factors including LDPE loading rates, cracking temperatures, and the type of zeolite catalysts used.
To analyze the process performance, four nature-inspired metaheuristic algorithms were applied: Gaussian Process Regression with Bayesian Optimization (GPR-BO), Artificial Neural Network with Particle Swarm Optimization (ANN-PSO), and two others. Initially, 13 input variables were considered, but those with a correlation coefficient below 0.49 were filtered out to refine the models. Feature selection techniques were employed prior to the modeling phase to enhance predictive accuracy.
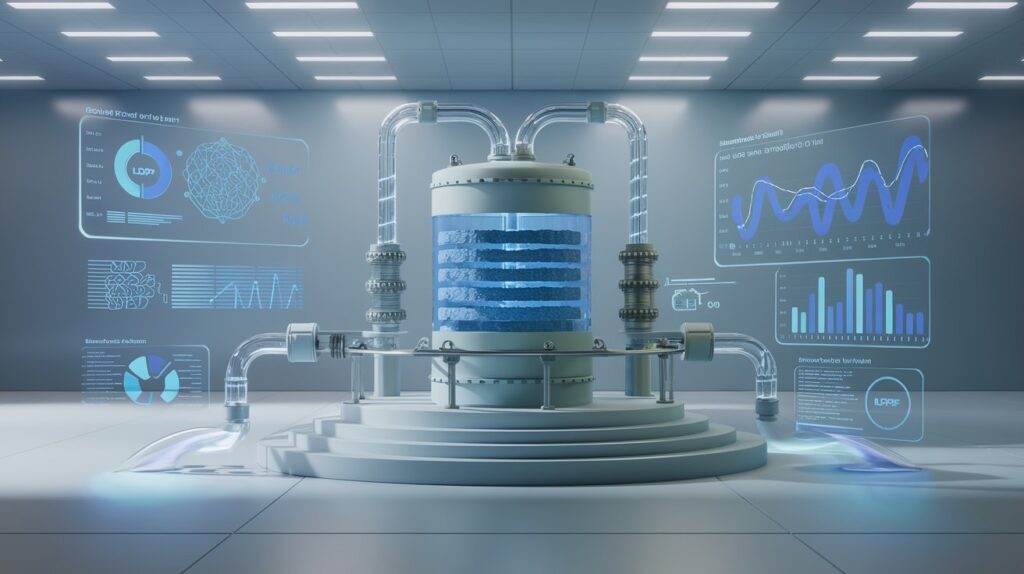
The models were evaluated using performance metrics such as Mean Square Error (MSE), Root Mean Square Error (RMSE), correlation coefficient (R), and coefficient of determination (R²). GPR-BO achieved the best performance in predicting HVGO/LDPE conversion, while ANN-PSO was most effective in forecasting naphtha yield. Both models achieved near-perfect accuracy levels, with R and R² values equal to 1.00 in training and testing datasets.
The findings demonstrate the potential of data-driven modeling combined with feature engineering for optimizing chemical processes in petrochemical industries. The approach not only contributes to waste reduction but also supports the development of more sustainable and efficient refining practices.
About the researcher
Dr. Abdullahi Garba Usman is a researcher at Faculty of Pharmacy and Operational Research Centre in Healthcare at Near East University. He specializes in chemical research, water analysis, chemometrics, and groundwater quality. His work bridges process modeling and environmental chemistry, with a strong interest in interdisciplinary approaches. He welcomes collaborative research opportunities and can be reached at abdullahigarba.usman@neu.edu.tr.
Abstract
The catalytic co-cracking of 2.5–10 wt% low-density polyethylene (LDPE) in heavy vacuum gas oil (HVGO) was carried out in a fixed-bed microactivity test (MAT) unit and studies on the effect of LDPE loading, cracking temperature and nature of zeolite catalyst generated reasonable data that were used to model HVGO/LDPE conversion and naphtha yield using four metaheuristic-based nature inspired optimization algorithms. Using 13 different input parameters into the data mining, less dominant variables with < 0.49 correlation co-efficient to the targets were filtered out. The effect of deterministic based feature on variable filtering prior to modeling stage was conducted for both HVGO/LDPE conversion and naphtha yield. The prediction performance of the developed models in both training and testing was evaluated using the mean square error (MSE), root mean square error (RMSE), coefficients of correlation (R) and determination (R2). Among the four algorithms, GPR-BO showed highest conversion prediction performance for both data training and testing, with MSE = 1.14 × 10−9 and 2.02 × 10−9, RMSE = 3.37 × 10−5 and 4.62 × 10−5, R2 and R = 1.00 respectively. For naphtha yield prediction, the ANN-PSO showed highest performance for both data training and testing, with MSE = 0.020 and 0.0663, RMSE = 0.143 and 0.189, R2 and R = 1.00 respectively.
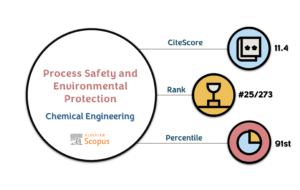
For further details, access the original paper from the publisher’s link: Optimization algorithms for modeling conversion and naphtha yield in the catalytic co-cracking of plastic in HVGO